Project Characteristics:
Usability Testing, Behavioral Finance, iMotions Platform (Facial Expression Analysis, Electrodermal Activity/Galvanic Skin Response)
Industry:
Finance
Project Description:
The goal of this case study is to share some best practices around measuring emotional engagement using the iMotions software platform. This case study is based on a user experience evaluation of an online tool called “Payback” (www.TimeForPayback.com) - an online game designed to educate students about the realities and consequences of the financial decisions they make in college (see Figures 1 and 2).
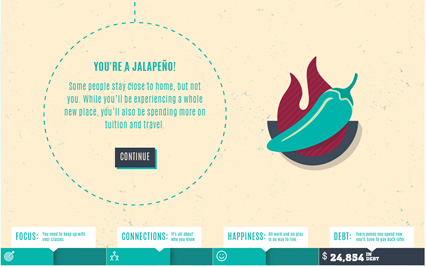
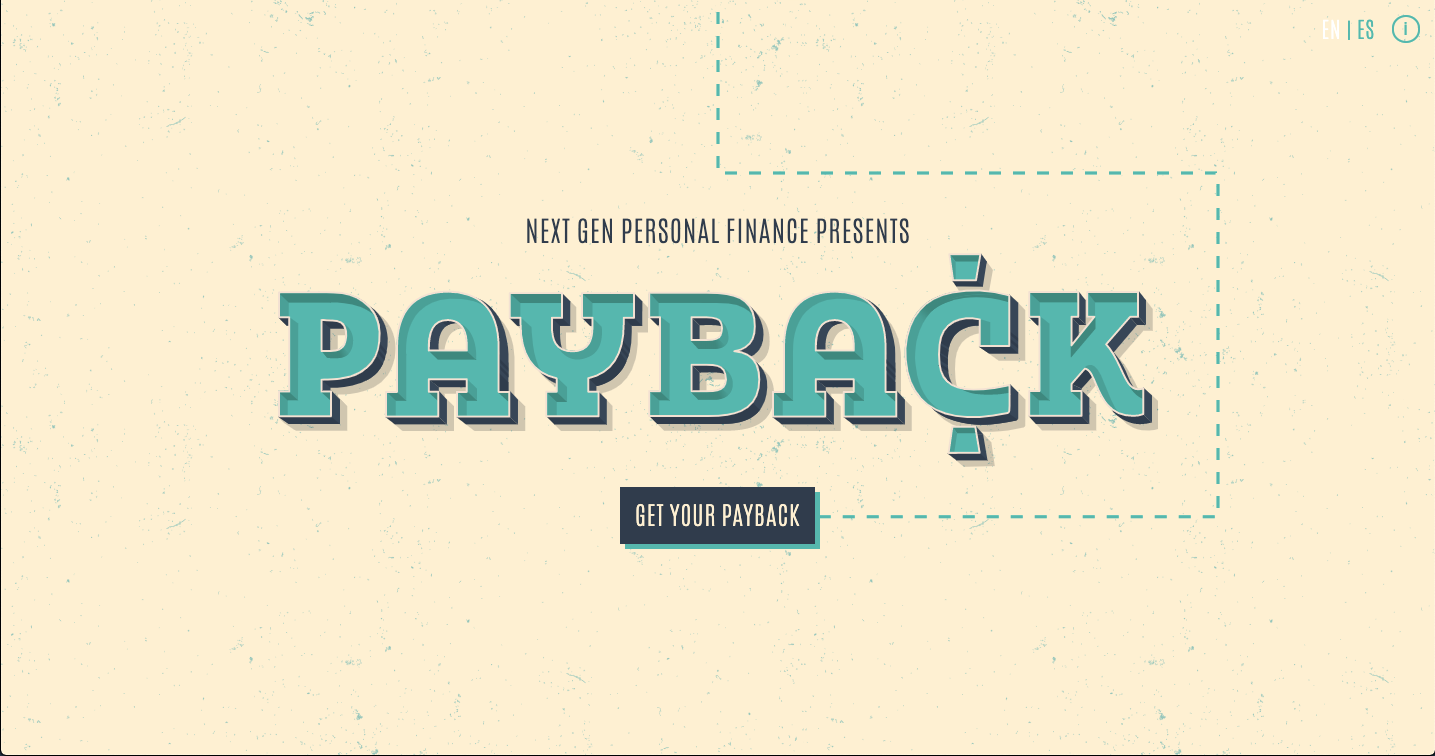
What We Did:
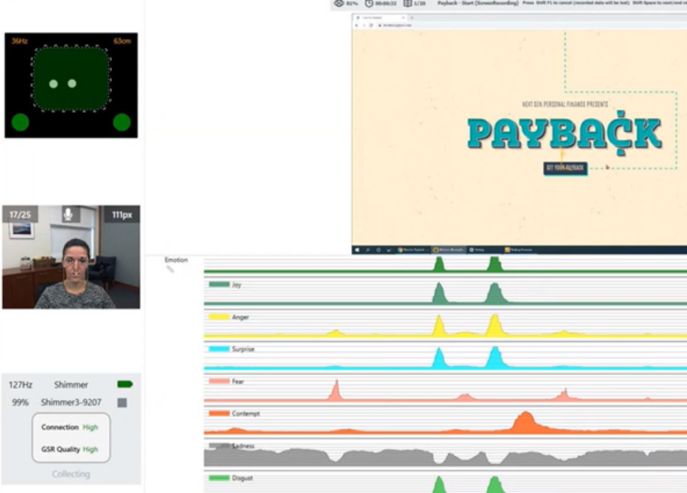
the iMotions platform.
We scheduled 60-minute interviews with 10 participants over four days, and during this time participants played two rounds of the personal finance game “Payback”. We gathered eye tracking and facial expressions (see Figure 3) via iMotions as well as Galvanic Skin Response (GSR) via a connected Shimmer device (see Figure 4). We also had participants self-report their emotions at set points during the game using the Self-Assessment Manikin Scale (SAM) (see Figure 5).
We made several adjustments to our study design to ensure accurate data collection:
- We had the participants play the game twice to mitigate variability in the learning process as participants learned from the game’s feedback.
- We adjusted our normal moderation style to make sure participants were not be speaking while biometric measurement was being taken.
- We paused our emotions tracking at set points of the game to ask participants to self-report their emotions based on the SAM scale.
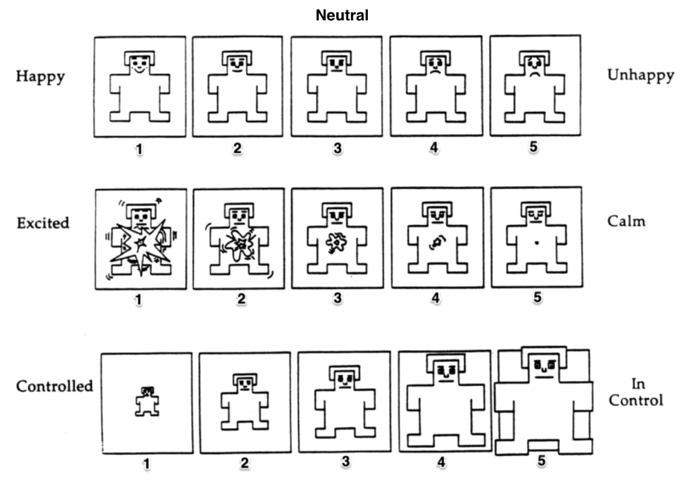
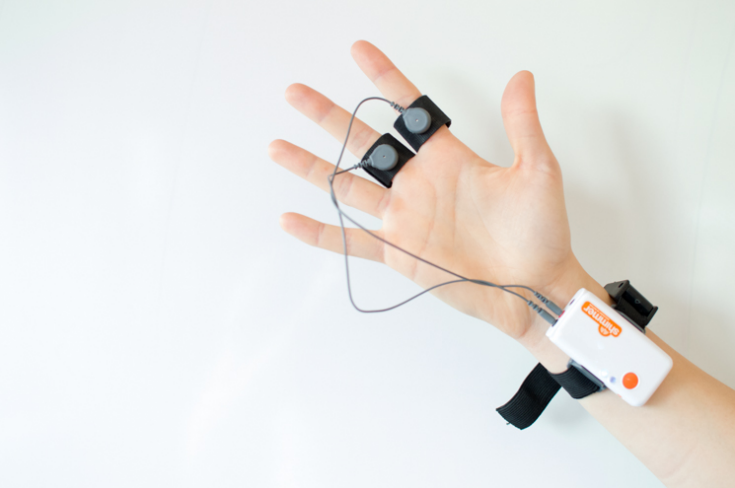
What We Found:
Our quantitative analysis found that collected GSR data loosely correlated with the SAM data (see figure 6 and 7), however, we found inconsistencies with our qualitative findings. Though participants self-reported they were happier, less excited, and more in control in Game 2, this feedback was not always consistent either the SAM or the iMotions data.
The results are consistent with previous research that says emotions are difficult to measure even across people in a single situation (Barrett et al., 2019). In our data analysis, we found that there was not great consistency between what a participant said, what they reported, and what iMotions measured. Specifically, we found that:
- Participants showed more arousal in higher peaks/min as measured by GSR in Game 2. A t-test showed that this difference was significant.
- Participants reported a difference in happiness (valence) between Game 1 and Game 2. A t-test showed that this difference was significant.
- Outside of GSR, there is no statistically significant difference in recorded emotions between Game 1 and Game 2.
- Participants self-reported they were happier, less excited, and more in control in Game 2.
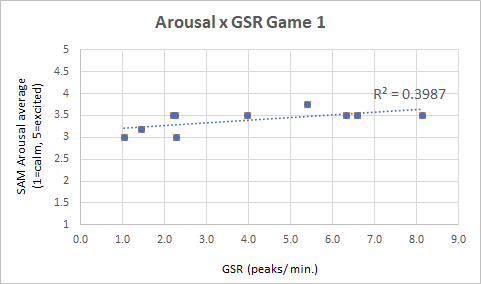
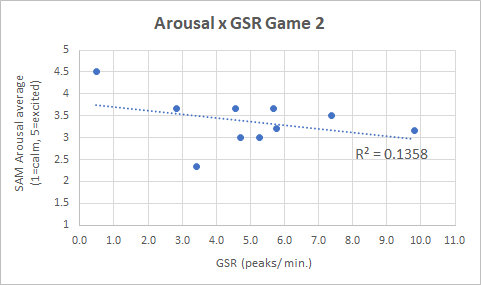
What Would We Do Different?
Of particular value from this study was the findings related to changes needed to be made for future iMotions studies:
- The design of the study should not include variability in the user flow. Tasks given should be as consistent as possible between users.
- The design of the study should limit verbal interaction between the participant and the moderator while facial expression data is being collected.
- This type of technology might be best suited for use in tightly constrained studies & linear development environments – e.g., movie trailers, A/B testing, and validation/summative studies involving still images or still screens.
The findings informed us on developing best practices that may be used by other UX professionals who may use biometric data and the iMotions platform for user related studies.
Best Practices for Measuring Emotional Engagement
Below are several best practices that were determined to achieve the most accurate results from the recorded biometric data and iMotions platform.
First, the design of the study should not include variability in the flow. For example, make sure that each participant:
- goes through the same tasks in the same order and
- sees the same content
Although the online game used in the study was a good demonstration to study behavior finance, data collection was difficult because participants didn’t have the same exact tasks. This made it challenging to compare entire participant sessions to each other because of the variability of experiences. Removing variability in the design of the study enables ease of data analysis. For this reason, gaming software isn’t recommended for these types of biometric studies because they have variable storylines or paths. This type of technology might be best suited for use in tightly constrained studies & linear development environments – e.g., movie trailers, A/B testing, validation/summative vs. formative studies involving still images or still screens.
Secondly, the design of the study should limit verbal interaction between the participant and the moderator while facial expression data is being collected. Practices like ‘think aloud’ invalidate results because it interferes with the emotion detection software. We mitigated this by pausing the recording at pre-determined points to allow the moderator to collect self-reported emotions. For these reasons, we can’t recommend this technology for studies where real-time think-aloud is a critical component. However, retrospective think-aloud (RTA) is a helpful option.
Lastly, emotion tracking software is a powerful new technology with various applications including testing two different versions of a website, reactions to an updated design, or other summative tests for example. Currently, it could best be used in summative or validation designs. A UX researcher could use emotion tracking software on formative or investigative research since it provides more data than would otherwise be available. Biometrics, in combination with the SAM provides opportunities to learn more, triangulate data and get a fuller picture to better answer the research questions.
Read our full whitepaper here.
References:
Barrett, L. F., Adolphs, R., Marsella, S., Martinez, A. M., & Pollak, S. D. (2019). Emotional Expressions Reconsidered: Challenges to Inferring Emotion From Human Facial Movements. Psychological Science in the Public Interest, 20(1), 1–68. https://doi.org/10.1177/1529100619832930
Contributions by Tyler Robert, Rachael Kelly, Natalie Wadia, Katharine Betteridge, Jacob Davidson, and Desmond Fang.